和合提供延伸服務(wù),成立涂裝服務(wù)團(tuán)隊(duì),為客戶(hù)提供量體裁衣式的、專(zhuān)業(yè)化的涂裝外包服務(wù)。
漆膜平整、豐滿(mǎn),光澤度高,耐性方面,耐濕熱、耐鹽霧,以及卓越的耐候性。同時(shí)具備高硬度高耐磨性、優(yōu)異的耐水性以及耐化學(xué)藥品性能。在潮濕或惡劣的氣候條件下漆膜仍能保持完整性和美觀(guān)性,顏色穩(wěn)定,不易變色,并且能夠抵抗外界因素的侵蝕。
防腐油漆配套需根據(jù)實(shí)際腐蝕環(huán)境進(jìn)行設(shè)計(jì)。一般大氣環(huán)境下,如C-1、C-2和C-3腐蝕環(huán)境,環(huán)氧防銹底漆和單、雙組分丙烯酸面漆即可,比較經(jīng)濟(jì)。C-4以上腐蝕環(huán)境,為重防腐體系。高鋅含量的富鋅底漆、云鐵中間漆和聚氨酯面漆為標(biāo)配,無(wú)論耐鹽霧還是耐老化性,要求極高。表中所列配套,皆能滿(mǎn)足不同腐蝕環(huán)境下的防腐蝕要求。
集裝箱對(duì)油漆的要求包括耐腐蝕性、耐磨性和柔韌性、耐候性、良好的施工性能、內(nèi)面漆的耐各種介質(zhì)性能,以及重涂性。性能穩(wěn)定可靠,具有良好的耐腐蝕性,能夠在-40℃至70℃的溫度范圍內(nèi)保持良好的防腐性能,保證正常使用5年。良好的耐磨性和柔韌性。良好的耐候性,能夠適應(yīng)廣泛的環(huán)境變化,保持色澤穩(wěn)定。
機(jī)械設(shè)備大多安置于室內(nèi),屬于C-3以下腐蝕環(huán)境,往往對(duì)外觀(guān)要求較高。如需更高要求,也可用脂肪族聚氨酯體系。底漆仍然采用雙組分環(huán)氧體系,附著力更優(yōu)。漆膜具有良好的硬度和韌性,以抵抗使用過(guò)程中的振動(dòng)、沖擊和摩擦。這對(duì)于確保油漆層不易脫落、劃傷或損壞至關(guān)重要。
鋼結(jié)構(gòu)配套需根據(jù)實(shí)際腐蝕環(huán)境進(jìn)行設(shè)計(jì)。一般大氣環(huán)境下,如C-1、C-2和C-3腐蝕環(huán)境,環(huán)氧防銹底漆和單、雙組分丙烯酸面漆即可,比較經(jīng)濟(jì)。C-4以上腐蝕環(huán)境,為重防腐體系。高鋅含量的富鋅底漆、云鐵中間漆和聚氨酯面漆為標(biāo)配,無(wú)論耐鹽霧還是耐老化性,要求極高。表中所列配套,皆能滿(mǎn)足不同腐蝕環(huán)境下的防腐蝕要求。
粉末涂料是一種新型的不含溶劑100%固體粉末狀涂料,具有無(wú)溶劑、無(wú)污染、可回收、環(huán)保、節(jié)省能源和資源、減輕勞動(dòng)強(qiáng)度和涂膜機(jī)械強(qiáng)度高等特點(diǎn)。?主要由?樹(shù)脂、?固化劑、?顏料、?填料和?助劑等組成,通過(guò)?靜電噴涂、?流化床浸涂法等方式涂覆于被涂物的表面,再經(jīng)過(guò)烘烤使其熔融流平,固化成膜。分為純聚酯型粉末、純環(huán)氧型粉末和環(huán)氧聚酯型粉末。
水性漆,顧名思義,是一種以水為稀釋劑的涂料,具有環(huán)保、安全、易清洗、干燥較快、具有良好的耐久性和廣泛的適用性等特點(diǎn)。
水性漆不含有害溶劑,對(duì)環(huán)境和人體健康無(wú)害。它使用水作為稀釋劑,減少了室內(nèi)空氣污染的風(fēng)險(xiǎn),同時(shí)也降低了火災(zāi)隱患,對(duì)施工人員的安全更有保障?。
水性漆不易燃,使用過(guò)程中不會(huì)產(chǎn)生火災(zāi)隱患,增加了施工過(guò)程中的安全性。
高固含涂料產(chǎn)品的特點(diǎn)主要包括高固體含量、低溶劑含量、環(huán)保性、高施工效率、良好的裝飾性以及特定的技術(shù)要求。高施工效率?:由于涂料固體分高,一道涂膜厚,從而大大提高了作業(yè)效率?。
良好的裝飾性?:高固含涂料具有與溶劑型涂料相同的裝飾性,能夠滿(mǎn)足各種美觀(guān)需求?。
高固含涂料以其環(huán)保、高效、高質(zhì)量的涂裝效果等特點(diǎn),在現(xiàn)代涂料市場(chǎng)中占據(jù)了一席之地。
單組份,可自然干燥,產(chǎn)生一定的硬度和附著力。但此時(shí)為“假干”,不是真正意義上的干燥。真正有機(jī)硅耐溫漆的干燥方式為高溫烘干。此類(lèi)漆在設(shè)備進(jìn)入使用狀態(tài)后,表面漆膜在運(yùn)行溫度下進(jìn)一步交聯(lián)干燥,此時(shí)漆膜擁有一定的耐水性、耐化學(xué)品性。
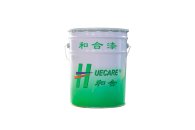
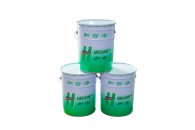
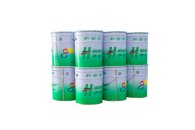
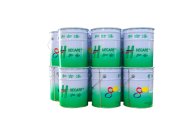
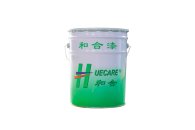
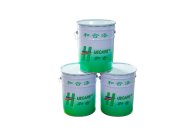
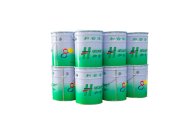
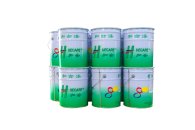
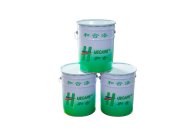
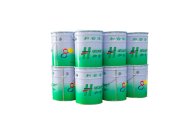











我們關(guān)愛(ài),我們分擔(dān),和合保護(hù)到永遠(yuǎn)
和合化學(xué)公司成立于2001年,公司致力于提供優(yōu)秀產(chǎn)品,提供優(yōu)秀服務(wù),培養(yǎng)優(yōu)秀人才,是您在油漆?涂裝行業(yè)的專(zhuān)業(yè)伙伴。公司擁有多種高新技術(shù)生產(chǎn)設(shè)備和高精尖檢測(cè)實(shí)驗(yàn)儀器設(shè)備,以及2200平方米的研發(fā)中心。
和合公司執(zhí)行嚴(yán)格的質(zhì)量及安全管理,與國(guó)內(nèi)外多家外知名企業(yè)、科研機(jī)構(gòu)及專(zhuān)業(yè)院校保持了良好的業(yè)務(wù)關(guān)系及技術(shù)交流,擁有先進(jìn)的生產(chǎn)工藝及技術(shù)配方。獲得ISO9001:2008質(zhì)量管理體系認(rèn)證......
了解更多
企業(yè)視頻
-
和合化學(xué)有限公司
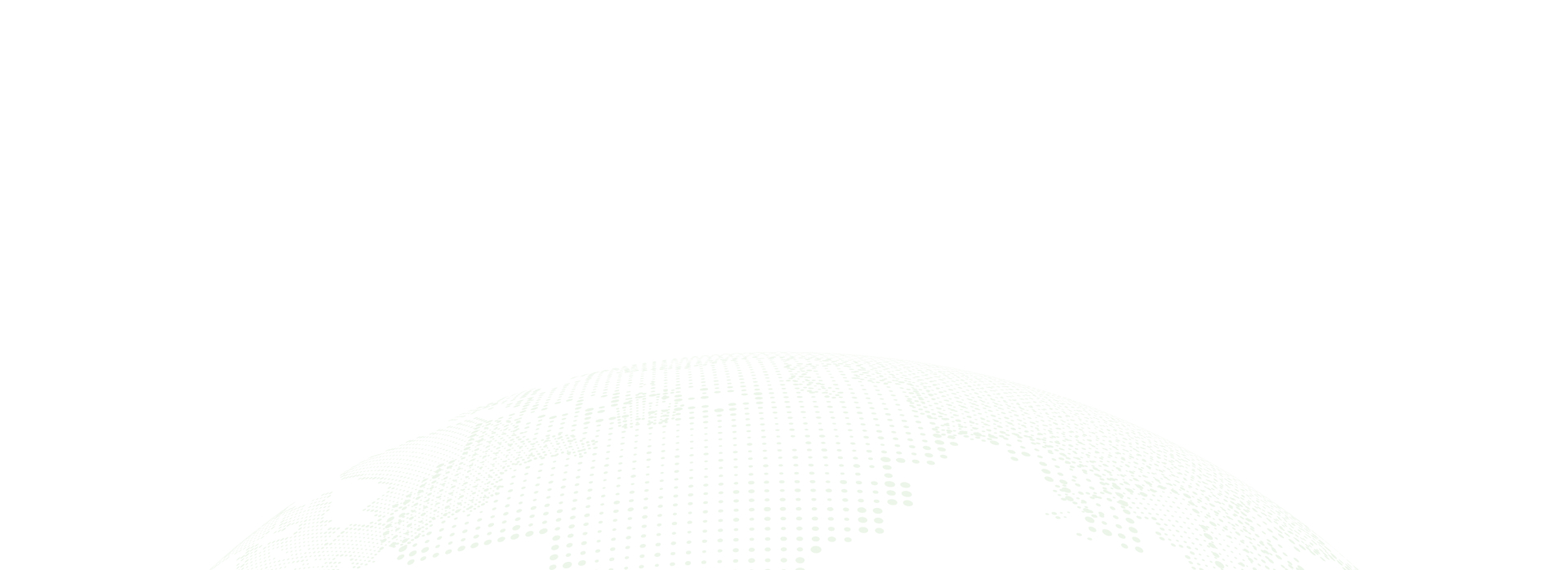
精細(xì)化、專(zhuān)業(yè)化、伙伴化,卓越品質(zhì)及時(shí)服務(wù)
-
青島國(guó)信體育場(chǎng)
-
青島地鐵口
-
青島海底隧道
-
龍口港
-
海陽(yáng)人工島大橋
-
青島膠州灣跨海大橋分段項(xiàng)目